How Big Data Analytics is Transforming Risk Management and Fraud Detection in Financial Services
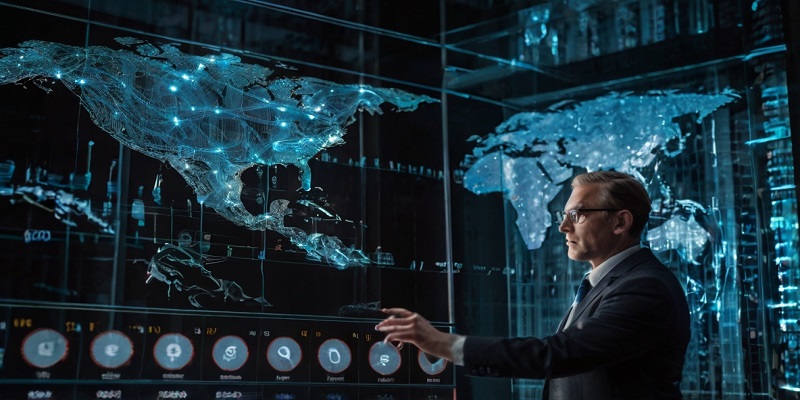
Risk management and fraud detection are critical components of maintaining stability and trust. Traditional methods have proven insufficient in addressing the complexities and speed of today’s financial landscape. Enter big data analytics, a powerful tool that is transforming how financial institutions approach these challenges. This article delves into the ways big data analytics is revolutionizing risk management and fraud detection in financial services, highlighting key benefits, technologies, and real-world applications.
The Role of Big Data in Financial Services
Understanding Big Data
Big data refers to vast volumes of data generated at high velocity from various sources, including transactions, social media, market data, and more. This data can be structured, semi-structured, or unstructured. The advent of big data has enabled financial institutions to collect, store, and analyze this information to gain valuable insights.
Importance in Financial Services
The financial industry generates enormous amounts of data daily. Utilizing big data analytics allows institutions to process this data efficiently, uncover patterns, and make data-driven decisions. Moreover, This capability is crucial for enhancing risk management and fraud detection processes, which rely heavily on accurate and timely information.
Transforming Risk Management
Predictive Analytics
Predictive analytics uses historical data to predict future events. In risk management, this means identifying potential risks before they materialize. By analyzing patterns in past data, financial institutions can forecast market trends, assess credit risks, and anticipate operational risks. This proactive approach helps in mitigating potential losses and making informed strategic decisions.
Real-Time Monitoring
Traditional risk management methods often involve periodic reviews and manual processes, which can be slow and reactive. Big data analytics enables real-time monitoring of transactions and market conditions. Advanced algorithms continuously analyze incoming data, providing immediate alerts when unusual patterns are detected. This capability allows financial institutions to respond quickly to emerging risks.
Enhanced Risk Assessment
Risk assessment involves evaluating the potential impact of various risk factors on an institution’s operations. Big data analytics enhances this process by incorporating a wider range of data sources, including social media sentiment, geopolitical events, and economic indicators. This comprehensive view enables more accurate risk assessments and better preparation for potential disruptions.
Revolutionizing Fraud Detection
Anomaly Detection
Fraud detection relies on identifying unusual activities that deviate from established patterns. Big data analytics excels in this area through anomaly detection techniques. Machine learning algorithms can analyze vast datasets to establish baseline behaviors for customers and transactions. In addition, when deviations from these norms occur, the system flags them for further investigation, reducing the incidence of undetected fraud.
Behavioral Analytics
Behavioral analytics focuses on understanding the behavior of individuals to detect potential fraud. By analyzing data such as transaction history, device usage, and location patterns, financial institutions can identify suspicious activities. For example, if a customer’s credit card is suddenly used in a different country shortly after being used locally, this could indicate fraud. Big data analytics makes it possible to detect these anomalies in real-time, preventing significant losses.
Network Analysis
Fraud often involves networks of individuals working together. Network analysis uses big data to identify relationships and connections between entities. By mapping out these networks, financial institutions can uncover hidden links and detect organized fraud schemes. This approach is particularly effective in combating money laundering and other sophisticated financial crimes.
Key Technologies Enabling Big Data Analytics
Machine Learning
Machine learning is at the heart of big data analytics. These algorithms learn from data and improve their accuracy over time. In financial services, machine learning models can predict risks, detect fraud, and automate decision-making processes. Thus, Their ability to handle vast amounts of data and identify complex patterns makes them invaluable tools.
Artificial Intelligence (AI)
AI goes beyond machine learning by incorporating elements like natural language processing and image recognition. In risk management, AI can analyze unstructured data such as news articles and social media posts to identify emerging risks. In fraud detection, AI can recognize visual patterns in documents and images that might indicate forgery or tampering.
Blockchain
Blockchain technology provides a secure and transparent way to record transactions. In the context of fraud detection, blockchain can create immutable records of transactions, making it difficult for fraudsters to alter data. Additionally, smart contracts can automate and enforce rules, reducing the likelihood of fraudulent activities.
Cloud Computing
Cloud computing offers the scalability needed to handle big data analytics. Financial institutions can store and process large datasets without investing in expensive infrastructure. Cloud-based analytics platforms provide the computational power required for real-time analysis, ensuring timely risk management and fraud detection.
Real-World Applications
Credit Scoring
Traditional credit scoring models rely on limited data points, such as credit history and income. Big data analytics expands this scope by incorporating alternative data sources like social media activity, online behavior, and transaction history. This holistic view allows for more accurate credit assessments, particularly for individuals with limited credit history.
Anti-Money Laundering (AML)
Money laundering involves disguising the origins of illegally obtained funds. Big data analytics enhances AML efforts by analyzing large volumes of transaction data to identify suspicious patterns. Machine learning models can detect anomalies and flag transactions that require further investigation, improving the efficiency of AML programs.
Market Risk Management
Financial markets are highly volatile, with prices influenced by numerous factors. Big data analytics helps institutions manage market risk by analyzing historical data and real-time market information. Additionally, Predictive models can forecast price movements, allowing traders to make informed decisions and hedge against potential losses.
Challenges and Considerations
Data Privacy
Handling large volumes of sensitive data raises privacy concerns. Financial institutions must ensure compliance with data protection regulations, such as GDPR and CCPA. Implementing robust data encryption and access controls is essential to protect customer information.
Data Quality
The accuracy of big data analytics depends on the quality of the data. Furthermore, Inconsistent or incomplete data can lead to incorrect conclusions. Financial institutions must invest in data cleansing and validation processes to maintain data integrity.
Integration with Legacy Systems
Many financial institutions still rely on legacy systems that may not be compatible with modern big data analytics platforms. Integrating these systems can be challenging and may require significant investment. A phased approach to integration can help mitigate disruptions.
Conclusion
Big data analytics is transforming risk management and fraud detection in financial services, offering unprecedented capabilities for identifying and mitigating risks. By leveraging technologies like machine learning, AI, blockchain, and cloud computing, financial institutions can enhance their predictive analytics, real-time monitoring, and anomaly detection processes. While challenges such as data privacy and integration with legacy systems exist, the benefits far outweigh the drawbacks. As big data analytics continues to evolve, its impact on financial services will only grow, ensuring a more secure and resilient financial ecosystem.